How can senior leaders in B2B SaaS companies leverage data to enhance their Go-to-Market (GTM) strategies and drive organizational growth?
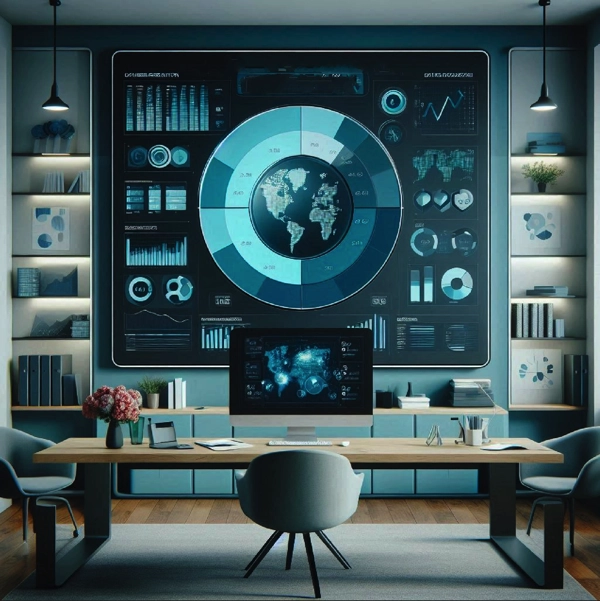
In today’s B2B SaaS environment, data has become a critical driver of strategic decision-making and competitive differentiation. The proliferation of digital channels and customer touchpoints generates vast amounts of information, offering insights that were previously inaccessible. Senior executives must leverage this data to inform their strategies, optimize operations, and anticipate market shifts. Harnessing the power of data is no longer a supplemental aspect of business—it is central to driving growth and sustaining a competitive edge.
A data-driven Go-to-Market (GTM) engine embodies the integration of advanced analytics into the core functions of sales, marketing, and customer success. By systematically collecting and analyzing data, organizations can refine customer segmentation, enhance targeting efforts, and improve conversion rates. This chapter serves as a guide for senior leaders aiming to develop a GTM engine that consistently yields data-backed decisions. It outlines practical steps to embed data-driven methodologies into organizational processes, enabling executives to respond swiftly to changing market dynamics and customer needs.
Transforming Go-to-Market Strategy with Data-Driven Account Segmentation
Data-Driven Account Segmentation is a critical component of an effective Go-to-Market strategy. In the GTM context, account segmentation involves categorizing potential and existing customers into distinct groups based on specific criteria such as industry, company size, purchasing behavior, and revenue potential. Advanced analytics enhance this process by sifting through vast datasets to identify high-potential accounts that align closely with the company’s value proposition. Proper segmentation enables organizations to allocate resources more efficiently, directing sales and marketing efforts toward segments with the highest likelihood of conversion and profitability. Achieving this level of precision necessitates a strong foundation of data quality, integration, and governance. Reliable and well-structured data ensures that segmentation is accurate and actionable, while robust governance practices maintain data integrity across the organization.
Predictive Opportunity Scoring leverages machine learning algorithms to assess and prioritize sales opportunities based on their likelihood of closing successfully. By analyzing historical data—including factors like lead source, engagement level, and past conversion rates—predictive models generate scores that help sales teams focus on the most promising deals. This approach not only improves conversion rates but also enhances deal efficiency by reducing time spent on less viable opportunities. Implementing predictive scoring requires collaboration between sales teams and data scientists to design models that accurately reflect the organization’s sales dynamics. The result is a more informed sales process where resources are allocated based on data-driven insights rather than intuition alone.
Real-Time Performance Dashboards offer decision-makers immediate visibility into key metrics across sales, marketing, and customer success functions. These dashboards consolidate data from disparate sources into unified visualizations, providing instant insights into areas such as lead response times, pipeline health, and win rates. Real-time data visualization empowers executives and managers to act swiftly in response to emerging trends or issues, facilitating more agile and responsive decision-making. By unifying various data sources, dashboards ensure that all stakeholders are operating with consistent and up-to-date information, which is crucial for aligning efforts across departments. The implementation of these dashboards requires thoughtful integration of tools and systems to ensure data accuracy and relevance for different organizational roles.
Improving GTM Success by Enhancing the Customer Segmentation Process
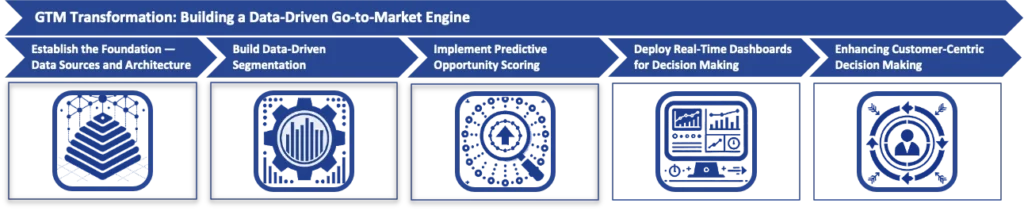
The process of building a data-driven Go-to-Market (GTM) strategy involves several structured steps. It begins by establishing a strong foundation of data sources and architecture, ensuring that data quality, integration, and governance are prioritized to create reliable insights. Next, the focus shifts to developing effective customer segmentation using both behavioral and firmographic data, supported by advanced analytics to refine customer profiles and target efforts more precisely. Predictive opportunity scoring is then implemented, leveraging historical data to rank opportunities based on their likelihood of success, which improves sales prioritization and efficiency. Real-time dashboards are deployed to provide decision-makers with instant access to key metrics, helping them make agile, data-driven decisions. Finally, organizations enhance their customer-centric decision-making by integrating qualitative and quantitative data to refine customer journey mapping and implement hyper-personalized outreach strategies, ultimately optimizing engagement and driving growth.
Establish the Foundation — Data Sources and Architecture
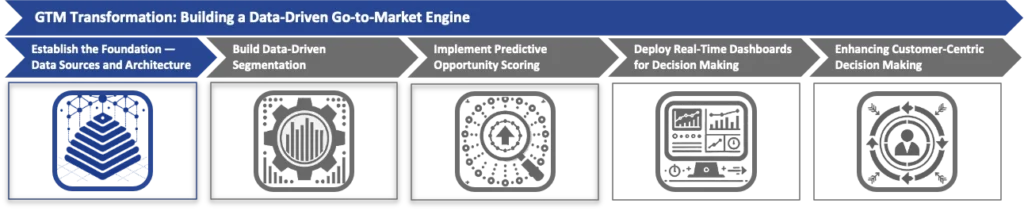
A robust data infrastructure is fundamental to any data-driven Go-to-Market strategy. Central to this foundation are the principles of data quality, integration, and governance. High data quality ensures that the insights derived are accurate and reliable, minimizing the risk of strategic missteps. Data integration is crucial for creating a unified view of information across various systems and departments, breaking down silos that can hinder collaboration and efficiency. Effective data governance provides the framework for managing data assets responsibly and establishing policies and procedures that maintain data integrity, security, and compliance with relevant regulations.
Identifying and implementing essential tools and systems is a critical step in building this foundation. Key technologies include Customer Relationship Management (CRM) systems, which track and manage customer interactions and sales pipelines, and marketing automation platforms that handle campaign management and lead nurturing. Additional tools might involve data warehousing solutions for storing and organizing large volumes of data, analytics platforms for processing and analyzing data sets, and data visualization tools that help interpret and present insights clearly. Selecting the right combination of technologies that align with the organization’s objectives and scale is vital for supporting data-driven initiatives.
Ensuring integration across departments enhances the ability to collect, clean, and centralize data effectively. This involves establishing interfaces and workflows that allow data to flow seamlessly between sales, marketing, customer success, and other relevant functions. Implementing data cleaning processes is essential to eliminate inaccuracies and redundancies, improving overall data quality. Centralizing data in a unified repository or through interconnected systems enables a holistic view of customer interactions and market trends. Such integration not only improves operational efficiencies but also facilitates more informed decision-making by providing stakeholders with access to consistent and comprehensive data sets.
Build Data-Driven Segmentation
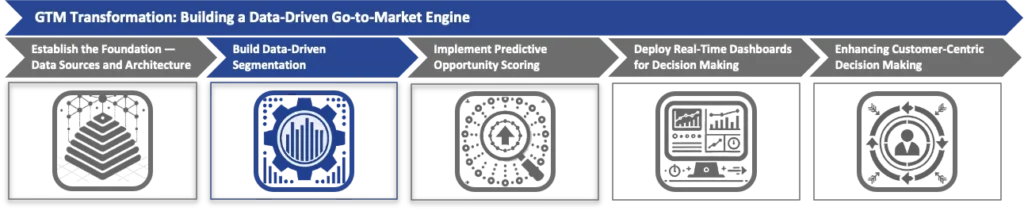
Developing effective customer segmentation is essential for tailoring Go-to-Market strategies to specific audience needs. Organizations should establish clear criteria for segmenting customers by analyzing behavioral data, demographics, and firmographics. Behavioral segmentation examines how customers interact with products or services, such as usage patterns or engagement frequency. Demographic factors might include job titles, roles within an organization, or geographic location. Firmographic segmentation focuses on organizational attributes like industry sector, company size, and revenue. By combining these dimensions, companies can create nuanced segments that enable more targeted and efficient marketing and sales efforts.
Leveraging data science techniques enhances the precision of customer personas and Ideal Customer Profiles (ICPs). Advanced analytics and machine learning algorithms can uncover patterns and correlations within large datasets that might be overlooked through traditional analysis. For example, clustering algorithms can group customers based on similarities in behavior or attributes, while predictive modeling can identify characteristics of high-value customers. This data-driven approach allows organizations to refine their customer personas and ICPs continually, ensuring they remain aligned with evolving market conditions and customer preferences.
Refining customer segmentation through data science not only improves targeting but also fosters better alignment between sales and marketing functions. With more accurate personas and ICPs, marketing teams can develop content and campaigns that resonate more effectively with each segment, while sales teams can prioritize leads that closely match the profiles of successful conversions. This alignment leads to more efficient resource allocation, higher conversion rates, and ultimately, a more effective Go-to-Market engine that is responsive to the specific needs of different customer groups.
Implement Predictive Opportunity Scoring
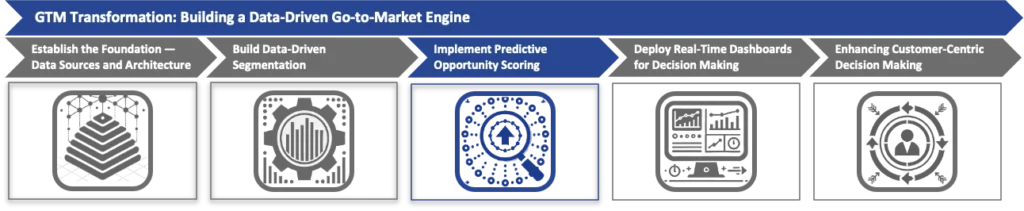
Implementing predictive opportunity scoring begins with a collaboration between sales leaders and data scientists to design a scoring model grounded in historical data. This data encompasses variables such as closed-won deals, lead sources, customer engagement levels, and sales cycle durations. By analyzing these factors, data scientists can identify patterns and key indicators that have historically led to successful conversions. This collaborative effort ensures that the scoring model is customized to reflect the unique sales dynamics and customer behaviors specific to the organization.
Automating deal prioritization based on the predictive insights derived from the scoring model is the next critical step. The model assigns likelihood scores to each opportunity, effectively ranking them according to their probability of conversion. Integrating this scoring system into the sales workflow enables sales teams to focus their efforts on high-priority deals with the greatest potential for success. Automation ensures that these scores are consistently updated as new data becomes available, allowing for real-time adjustments in sales strategies and resource allocation.
Implementing predictive opportunity scoring not only enhances the efficiency of the sales process but also contributes to more accurate forecasting and improved conversion rates. Sales managers gain a data-driven tool for coaching and performance management, while individual sales representatives benefit from clear guidance on where to concentrate their efforts. Over time, the predictive model can be refined and adjusted based on its performance, creating a feedback loop that continually improves the organization’s ability to prioritize opportunities effectively.
Deploy Real-Time Dashboards for Decision Making
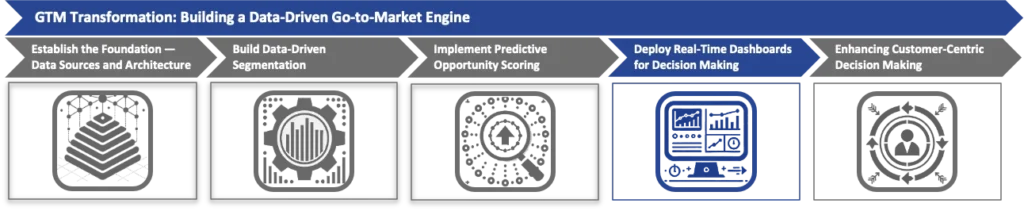
Deploying real-time dashboards is a critical step in fostering a data-driven decision-making culture. The effectiveness of these dashboards heavily relies on the underlying data quality, integration, and governance. High-quality data ensures that the insights drawn are accurate and actionable, while seamless integration consolidates information from various sources—such as CRM systems, marketing automation platforms, and customer service tools—into a cohesive view. Robust data governance policies maintain data integrity and security, establishing trust in the analytics provided.
Creating dashboards tailored to specific organizational roles enhances their relevance and utility. Executives may require high-level overviews featuring metrics like total sales revenue, market growth, and strategic KPIs. Sales teams might focus on lead response times, pipeline progression, and individual win rates. Marketing professionals could benefit from insights into campaign performance, lead generation efficiency, and conversion metrics. By customizing dashboards to address the unique needs of each department, organizations ensure that stakeholders have immediate access to the most pertinent information for their responsibilities.
Training teams to interpret and act on dashboard insights is essential for embedding data-driven practices into daily operations. This involves educating employees on not only how to navigate the dashboards but also how to analyze the data to make informed decisions. Encouraging regular use of these tools helps teams to identify trends, recognize areas for improvement, and make timely course corrections. By fostering an environment where data informs strategy and execution, organizations can enhance agility and responsiveness in a rapidly changing market landscape.
Enhancing Customer-Centric Decision Making
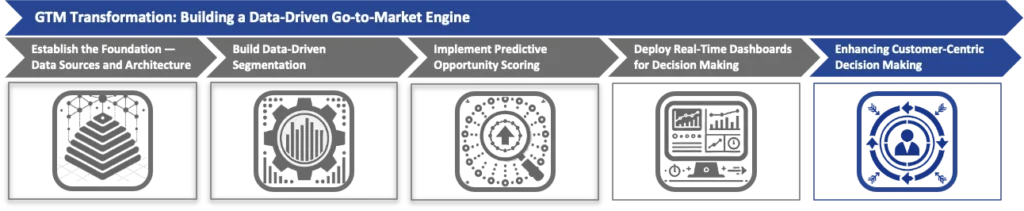
Enhancing customer-centric decision-making involves leveraging data to sharpen the focus on customer needs across all Go-to-Market activities. The objective is to ensure that every strategic and operational decision reflects an in-depth understanding of customer preferences and requirements. By integrating diverse data sources, organizations can develop a holistic view of their customers, leading to more targeted marketing efforts and more effective product development strategies.
One actionable step is Customer Insights Integration, which entails combining qualitative customer feedback with quantitative data insights. This integration might involve analyzing customer satisfaction surveys alongside product usage analytics to uncover nuanced insights into customer behaviors and needs. By forming a comprehensive understanding of the customer, organizations can tailor their marketing and product development efforts more precisely, addressing specific pain points and preferences that drive customer engagement.
Another critical action is Customer Journey Mapping using real-time data from dashboards and predictive scoring. By continuously refining and optimizing customer journey maps, companies can align their strategies with touchpoints where improvements or proactive engagement can make a significant difference. Additionally, implementing Hyper-Personalization Initiatives allows organizations to develop campaigns based on data-driven segmentation. By tailoring content, offers, and outreach strategies to individual customer segments—and leveraging predictive insights to anticipate needs—companies can maximize engagement and retention, fostering stronger customer relationships.
TechAdapt’s GTM Transformation From Reactive to Predictive
TechAdapt, a mid-sized B2B SaaS company specializing in workflow automation solutions, faced stagnation in its sales growth despite a robust product offering. Recognizing the limitations of their traditional sales approach, the executive team decided to transition to a data-driven Go-to-Market engine. They began by adopting predictive opportunity scoring and collaborating with data scientists to design a model that analyzed historical data such as closed-won deals, lead sources, and engagement metrics. This scoring system enabled the sales team to prioritize deals with the highest likelihood of conversion, focusing resources where they were most likely to yield results.
In parallel, TechAdapt refined its account segmentation using advanced data insights. By analyzing behavioral patterns and firmographic information, they identified high-potential customer segments that were previously overlooked. The marketing team leveraged this segmentation to tailor campaigns more effectively. Additionally, the company implemented real-time performance dashboards that unified data from sales, marketing, and customer success departments. These dashboards provided executives and team leaders with immediate visibility into key metrics, enhancing decision-making during weekly sales meetings. As a result of these integrated efforts, TechAdapt experienced a 15% increase in win rates and a 20% improvement in lead qualification accuracy, positioning them for sustained growth in a competitive market.
Integrating Key Components for a Cohesive Data-Driven GTM Strategy
In summary, developing a data-driven Go-to-Market (GTM) engine hinges on integrating key components such as data-driven account segmentation, predictive opportunity scoring, and real-time performance dashboards. These elements work in tandem to create a cohesive GTM strategy that enhances efficiency and alignment across sales, marketing, and customer success functions. By establishing a robust data infrastructure and integrating disparate data systems, senior leaders can build advanced predictive models and deploy dashboards that facilitate informed decision-making at every organizational level.
For successful implementation, executives must foster cross-functional alignment and cultivate a data-driven culture within their organizations. This involves not only investing in the right technologies but also encouraging a mindset where data literacy and evidence-based decision-making are embedded in daily operations. Emphasizing customer-centric decision-making ensures that strategies remain aligned with evolving customer needs, leading to deeper engagement and higher retention rates. Ultimately, adopting a structured, step-by-step approach to building a data-driven GTM engine offers strategic benefits such as improved revenue forecasting, optimized sales cycles, and a significant competitive advantage in the marketplace.